Voice trigger detection plays a crucial role, which enables voice assistant activation when a target user says a keyword phrase. A detector is typically trained on speech data independent of speaker information and used for the voice trigger detection task. A speaker-independent voice trigger detector, however, often performs worse on a speech from underrepresented groups.
In this blog, we discuss a novel voice trigger detector model that can increase detection accuracy by using a limited number of utterances from a target speaker. This voice trigger detection model uses an encoder-decoder architecture in contrast to traditional detectors, which conduct speaker-independent voice trigger detection, and metric learning-trained decoders anticipate a unique embedding for each syllable. By adjusting to the target speaker's speech when calculating the voice trigger score, the tailored embedding increases the precision of voice trigger detection.
How to improve Voice Trigger Detection using metic learning?
As discussed above, to improve voice trigger detection using metric learning we will be using an encoder & decoder.
A speaker-independent phoneme prediction is performed by an encoder while a decoder provides speaker-adapted voice trigger detection in the proposed MTL method for enhancing voice trigger detection using metric learning.
Down below we will understand the functions of Encoder and Decoder separately in detail.
Encoder
The encoder is made of N stacked Transformer encoder blocks with self-attention. The phoneme predictions carried out by the self-attention encoder convert the input feature sequence, represented by X, into hidden representations as:
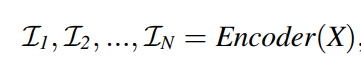
where IN denotes a hidden representation after the n-th encoder block. In order to get logits for phoneme classes, a linear layer is applied to the final encoder output IN.
Decoder
Since the speaker information might be reduced at the top encoder layer, we utilise an intermediate representation (n<N). The trainable vectors are denoted by {qm||m = 1, ..., M} where qm ∈ Rdx1. The encoder output and the query vectors are fed together to produce a set of decoder embedding vectors as

The output of the P-stacked Transformer decoder block is indicated by em ∈ Rdx1. The decoder output set is then modified. to create a size dM × 1 utterance-wise embedding vector.
Then, two task-level linear layers are branched at this point: First, the embedding is covered with a linear layer to forecast a scalar logit for the keyword phrase. The second linear layer is added for speaker verification and obtaining logits. Additionally, we employ the decoder embedding and metric learning is carried out in a mini-batch.
Multi-task learning
We first introduced the MTL framework for keyword spotting, and now we propose the metric-learning loss to compare the decoder embeddings and provide a speaker-adapted speech trigger detection score. The model is trained using phonetic loss in the MTL framework at the encoder output. There are three branches available at the decoder output: speaker identification, keyword-phrase loss, and metric learning loss.
The following can be used to formulate the training's objective function:

where the terms L(phone), L(spkr), L(phrase), and L(metric) stand for the respective phonetic loss, speaker-identification loss, keyword-phrase loss, and metric learning loss. The scaling variables for balancing the losses are α, β, and γ.
L(phone)is a phonetic loss to compute a voice trigger detection score, L(phrase) is a loss due to cross-entropy on the scalar logits, and L(spkr) is a speaker cross-entropy loss.
The metric loss L(metric) is a cosine similarity metric. For positive pairings, referred to as utterances, the decoder embedding output is directly affected by scale and offset settings. Defines as utterances from the same speaker that contain the keyword phrase, and the negative pairings represent utterances from other speakers or utterances from the same speaker with phrase labels that are the reverse of the keyword phrase. The cosine similarity is first turned into a probability as :
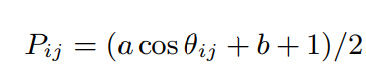
where cosθij is the cosine distance between the i-th and j-th utterances' decoder embeddings. The parameters a and b stand for trainable scale and offset parameters, respectively. One may calculate Loss in metric L(metric) as:

where NP and NN represent the numbers of positive and negative pairings, and P and N represent sets of the positive and negative pairs inside a mini-batch.
Training of the model
For the purpose of training the MTL tasks, two sources of data are employed every mini-batch. The first source, which is mostly utilised for phonetic loss and keyword phrase loss, is a collection of anonymized utterances with either phoneme labels or keyword phrase labels (voice trigger data).
The voice trigger data's non-keyword utterances are also employed as a negative class measure of learning loss. Combining an ASR dataset with the phoneme labels with a keyword spotting dataset with the keyword phrase labels will provide the dataset. The second set of data consists of speaker-labelled utterances (speaker-ID data), where each utterance is composed of a keyword phrase and a non-keyword sentence.
For each mini-batch of training, a batch sampling method is used to choose samples from each of these sets. For a batch size of 128, for instance, we choose 112 utterances from the speaker-ID data, which comprises 4 utterances from 28 different speakers, and the remaining utterances come from the voice-trigger data. In order to establish negative pairings (keyword vs. non-keyword) for the same speaker and aid in metric learning, the keyword phrase segment is also omitted at random for the utterances selected from the speaker data.
Similarity score
When making an inference, we begin by obtaining an anchor embedding, which is the average of the decoder embeddings from the speaker's prior utterances. The test utterance's decoder embedding is then calculated.
We then determine how similar the test embedding and anchor embedding are. The speaker-adapted voice trigger score and the similarity score line up. The speaker-adapted score and a speaker-independent voice trigger score Sctc produced from the encoder output can optionally be combined.
The speaker-adapted score is calibrated first as follows: Smetric = (Pi, anchor − C)/D, where C and D are the global parameters, mean and standard deviation. Next, we integrate the results using a simple weighted average to combine these two voice trigger scores:

here µ is the weighting factor.
Conclusion
In this blog, we explored a cutting-edge method for enhancing speech trigger detection by metric learning speaker information adaptation. The encoder conducts phoneme prediction for a speaker-independent voice trigger detection while the decoder predicts an utterance-wise embedding for a speaker-adapted voice trigger detection in this metric learning architecture. The decoder embedding for a test utterance and the anchor embedding for each speaker is compared to get the speaker-adapted voice trigger score.
According to the results, metric learning surpasses the speaker-independent speech trigger detector as a baseline by 38% in terms of FRRs.