What are the three important types of neural networks in AI that are regularly used and are mostly talked about? Let’s scroll more on how they operate, and how they are utilized.
The three most important neural networks are:
- ANN (Artificial Neural Network)
- CNN (Convolutional Neural Network)
- RNN (Recurrent Neural Network)
First, let's start with a quick explanation of what neural networks in AI are.
What is a Neural Network in AI?
A neural network is a network or circuit made up of biological neurons, or, in a more contemporary meaning, an artificial neural network made up of artificial neurons or nodes. Thus, a neural network can be either a biological neural network (made up of biological neurons) or an artificial neural network in AI, made of nodes (used to solve Artificial Intelligence issues). Artificial neural networks mimic biological neuron connections as weights between nodes.
Neural Networks in AI can discover hidden patterns and correlations in raw data using algorithms, cluster and categorize them, and learn to improve over time.
However, just as different tasks need different understandings, neural networks are no exception. While a certain type of neural network might outperform for a specific type of problem, it may even underperform if applied to some other type of problem.
That is why the classification of neural networks is done, to optimize the results by using the right neural network for different use cases.
ANN vs CNN vs RNN-
There are hundreds of neural networks available to handle issues throughout many domains. In this section, we'll go through the classification of neural networks as ANN vs CNN vs RNN.
ANN Artificial Neural Network-
ANN learning has been effectively used to learn real-valued, discrete-valued, and vector-valued functions containing challenges such as analyzing visual scenes, voice recognition, and learning robot control techniques.
ANNs send data in one direction, passing it through multiple input nodes until it reaches the output node. Due to this, ANNs are also known as Feed-Forward networks. Hidden node layers may or may not exist in the network, making its operation more understandable and making ANNs one of the most basic neural network versions.
In ANNs, a problem might have numerous instances, each of which is represented by a set of attribute-value pairs. ANNs used to solve issues with a target function output can be discrete, real, or a vector of many real or discrete-valued properties. ANN learning methods do not have an issue with noise in the training data. There may be faults in the training samples, but they will have no effect on the final result. It is commonly utilized in situations when a quick assessment of the learned target function is necessary. The total number of weights in the network, the total number of training instances evaluated, and the settings of different learning algorithm parameters can all contribute to extended training durations for ANNs.

What should ANNs be used for?
The ANN is employed in technology that focuses on difficult issue solving, such as pattern recognition challenges.
Here are several examples:
- For business intelligence, predictive analysis is used.
- A speech-to-text transcription program that converts spoken words into text.
- Recognition of handwriting and facial expressions.
- Email spam detection.
- Forecasting the weather.
Limitations of ANNs-
- ANNs are capable of working only with numerical data. Before being brought to ANN, problems must be transformed into numerical values.
- Experience and trial and error are used to create the ideal network structure as the structure of artificial neural networks are determined by no explicit rule.
- The trust in ANN is low as when ANN provides a probing answer, it does not explain why or how it was chosen.
CNN (Convolutional Neural Network)
A Convolutional Neural Network (ConvNet/CNN) is a Deep Learning system that can take an input picture, assign relevance (learnable weights and biases) to different aspects in the image, and distinguish between them. Or in other words, the CNN's job is to compress the pictures into a format that is easier to manage while retaining key components for generating a good forecast
This neural network computational model employs a multilayer perceptron variant and includes one or more convolutional layers that can be linked or pooled altogether. These convolutional layers provide feature maps that capture a portion of an image, which are then split down into rectangles and routed to nonlinear processing.
The first ConvLayer is in charge of collecting low-level details like edges, color, gradient direction, and so on. The design changes to the High-Level properties as well with the addition of layers, giving us a network that understands the photographs in the dataset in the same manner, as we do.
A CNN requires substantially less pre-processing than other classification algorithms. Whereas simple approaches require hand-engineering of filters,
With adequate training, CNNs can learn these characteristics.
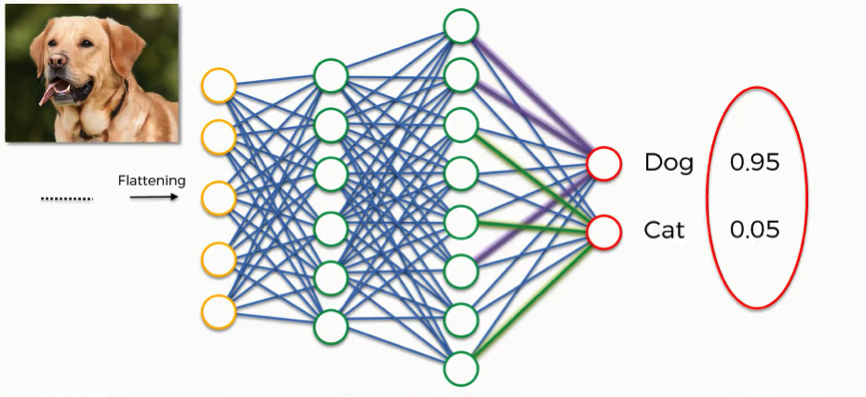
What should CNNs be used for?
The most productive use for CNNs is image classification, for eg:- Labeling hand-written letters and digits or identifying satellite images that contain roads.
Limitations of CNNs
- CNNs do not encode the position and orientation of objects, therefore if the pictures have a degree of tilt or rotation, CNNs have a hard time categorizing them.
- Inability to be spatially invariant with respect to the supplied data.
- Coordinate frames, which constitute an essential component of human vision, are not present in CNNs.
- A ConvNet requires a large dataset to process and train the neural network.
.
RNN(Recurrent Neural Network)
RNNs make use of sequential data, such as time-stamped data from a sensor device or a spoken speech made up of a series of words. Unlike standard neural networks, a recurrent neural network's inputs are not independent of one another, and each element's output is reliant on the computations of the elements before it, the output from the previous phase is supplied into the current stage as input.
RNNs have a "memory" that stores all information about the calculations. Because it delivers the same result by doing the same job on all inputs or hidden layers, it uses the same parameters for each input. Unlike other neural networks, the complexity of the parameters is reduced.
RNNs are utilized in applications such as forecasting and time series analysis. With recurrent neural networks, even convolutional layers are used to extend the effective pixel neighborhood.
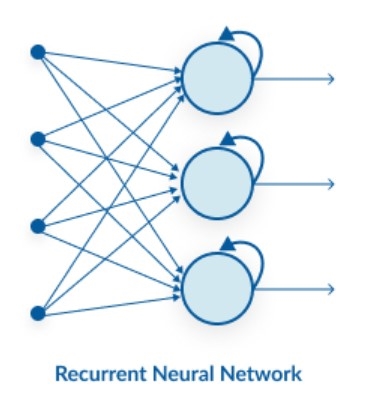
What should RNNs be used for?
RNN can produce pretty exact predictions since it has internal memory. Furthermore, it may be used to solve problems with sequential data.
In light of this, RNN applications include:
- Prediction issues Automated translation
- Speech recognition
- Analysis of public sentiment
- Forecasting stock prices
- Text generation and language modeling
Limitations of RNNs
- The problem of disappearing or exploding gradient RNNs can't be stacked.
- RNNs are recurrent, which means that training them will take a long period.
- When compared to feedforward networks, the overall training pace of RNN is rather slow.
- It's more difficult to process longer sequences.
The Crux
So, what are you working on?
If you're just getting started with Machine Learning, it's critical to understand and identify the problem you're attempting to address.
Remember:
- Artificial Neural Networks (ANNs) are useful for resolving complicated issues.
- CNNs (Convolutional Neural Networks) are the most effective way to solve computer vision issues.
- RNNs (Recurrent Neural Networks) are capable of processing natural language.